Artificial intelligence dominates much of practically every space in technology, including Nvidia CEO Jensen Huang’s keynote at CES 2025. The technology will be integral to the company’s GeForce RTX 50 Series, more so than any generation of graphics cards prior. This should come as no surprise, given how much existing stock enhances applications through machine learning, creative and gaming alike.
As the wait to see how GeForce RTX 50 Series will push performance envelopes grows shorter, I feel it’s important to assess where things stand at present. It’s easy to forget, but the GeForce graphics cards that make up almost 90% of the market are capable of more than rendering pretty pixels and frames. In fact, there’s a wealth of ways they can enhance creative and productive workflows by flexing their considerable AI chops.
The Tensor Cores of RTX AI
First, a quick history lesson with a sprinkle of maths. Back in 2018, GeForce RTX 20 Series became the first consumer graphics cards family to feature specialised hardware accelerators for ray tracing and artificial intelligence. You may know them better by ‘ray tracing cores’ and ‘Tensor Cores’, respectively, working in tandem with Nvidia’s long-established CUDA and shader cores.
Tensor Cores derive their name from their ability to process their namesake: tensor mathematics. Without getting stuck into the weeds of what a tensor is, all you need to know is they’re paramount in the application of machine learning. Without them, processing the countless variables of neural networks would be practically impossible.
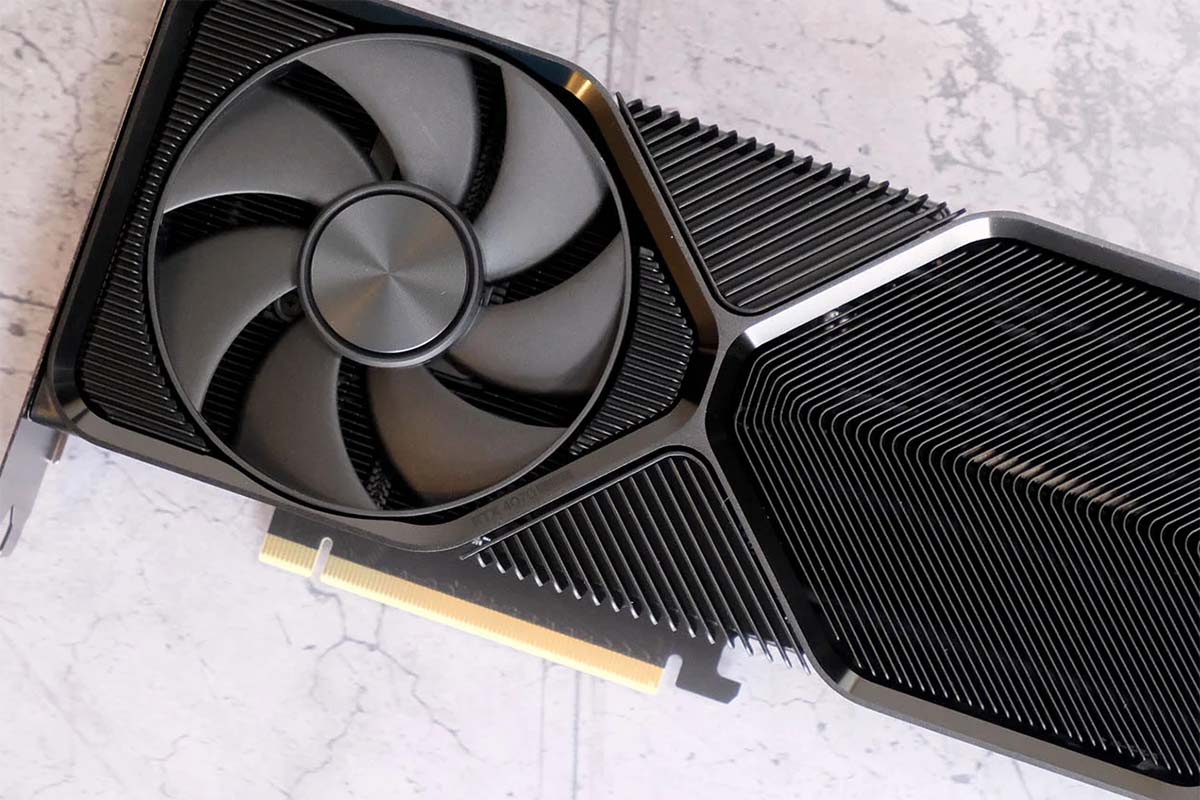
It’s for this reason that Tensor Cores are the driving force behind much of Nvidia’s DLSS technology. These cores do the vast majority of the heavy mathematical lifting required to upscale images and increase frame rates, oftentimes with the output actually better than the native resolution because of the intelligent way in which DLSS improves visual fidelity through machine learning. It’s not magic, but it’s close.
Upcoming GeForce RTX 5090 raises the bar to 3,352 TOPS – an incomprehensible 9,211% increase over NPU-type processors.
However, crucial to the burgeoning army of content creators, these Tensor Cores are also capable of providing AI-enhanced experiences outside of gaming. In the latter arena, it’s possible to measure their performance in AI TOPS (Trillion Operations Per Second). The same TOPS are also available from other common accelerators such as the CPU or GPU, albeit at throughput that’s magnitudes lower. It’s this relative slowness that makes these accelerators unsuitable for gaming environments, where all the required work needs to be accomplished in milliseconds.
To put GeForce TOPS hegemony into context and using the best CPUs as a benchmark, the Tensor Core NPU in latest desktop Intel Core Ultra 9 285K hits a peak of 36 INT8 TOPS while Intel Core Ultra 9 288V rules the laptop roost with 120 TOPS achieved by its combination of CPU, GPU, and NPU. By comparison, Nvidia is well ahead of its competitors, with the upcoming GeForce RTX 5090 raising the bar to 3,352 TOPS – an incomprehensible 9,211% increase over accompanying NPU-type processors.
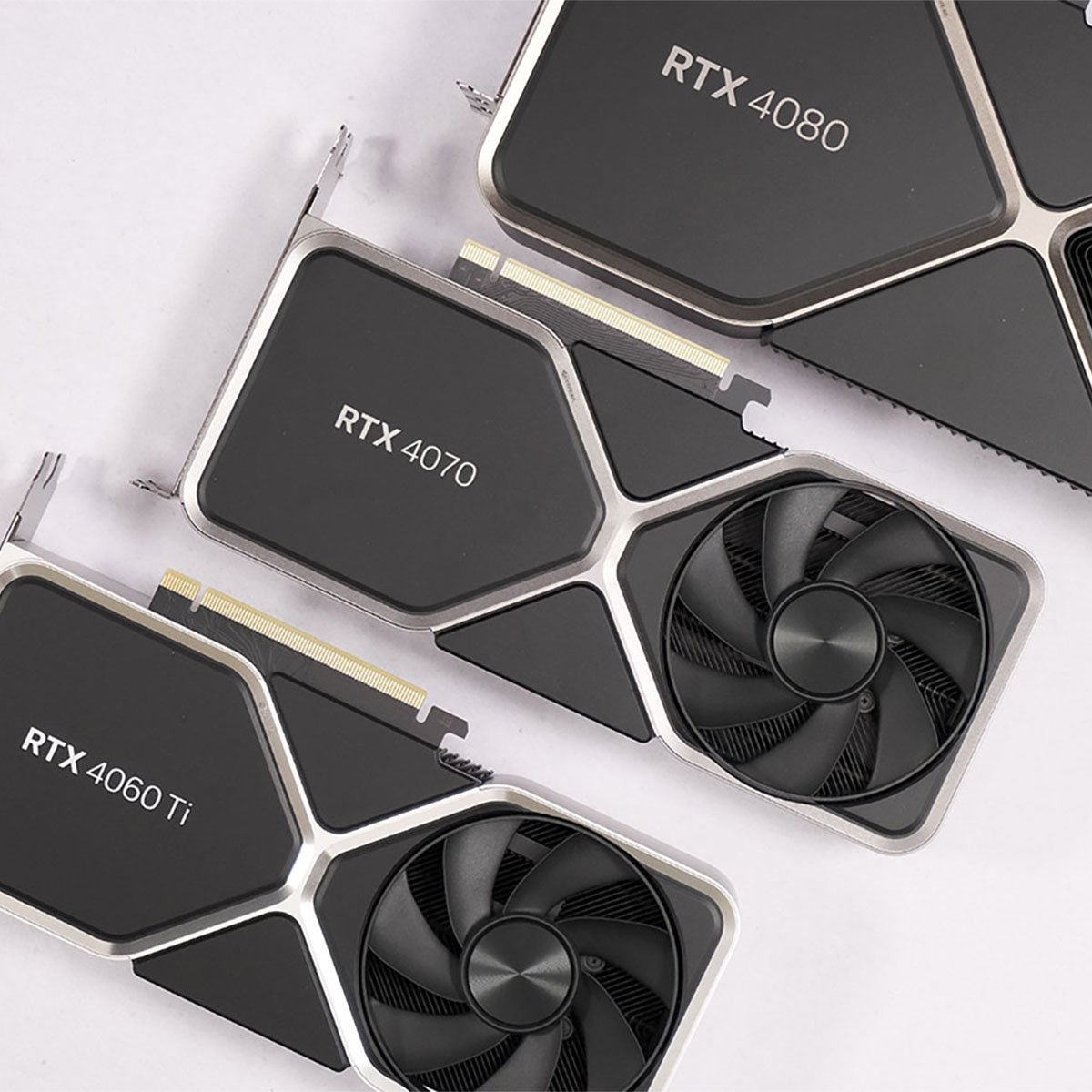
This isn’t a new story, as Nvidia’s unified architectural approach puts its consumer cards in line with enterprise alternatives. Team Green had its current rivals beat back in 2020 with its Ampere-driven GeForce RTX 3070 running 166 TOPS. Meanwhile, its flagships run away with monumental uplifts, as RTX 4090 pushes the boundaries to 1,321 AI TOPS courtesy of 512 Tensor Cores and RTX 5090 has another 2.5x gain on top of that.
…there’s an enormous amount of performance to extract from the Tensor Cores in our GeForce RTX graphics cards.
Suffice to say, there’s an enormous amount of performance to extract from the Tensor Cores in our GeForce RTX graphics cards. This hasn’t gone unnoticed by software vendors, with giants like Adobe and more updating their applications with features that specifically call upon AI acceleration made possible by well-optimised, fast Tensor Cores.
Tensor Cores are the bedrock of running fast, accurate generative AI and processing large-language models such as ChatGPT. It’s no stretch to say that without them, many of the wow-inducing applications you take for granted today would simply not be around. Of course, Nvidia has also developed a few tools in its lab that are worth checking out and make for a great starting point.
QoL Improvements
You don’t need to be a creative professional to benefit from the advantages GeForce RTX AI offers. In fact, Nvidia offers several applications that you can check out for free via its website with minimal fuss.
ChatRTX
Chances are you’re familiar with chatbots like ChatGPT or other cloud-based Large Language Models (LLMs) such as Microsoft Copilot. However, it is possible to run a personalised LLM on your PC using the grunt of your graphics card. Case in point, ChatRTX.
Using ChatRTX, you can query a local dataset such as a project brief or your personal photo collection to aid with summarisation and search. There’s no need for an internet connection to do this, as everything happens right on your system courtesy of a compatible GeForce RTX GPU.
Running ChatRTX in place of a cloud-based LLM comes with advantages to security and latency. Any materials fed into ChatRTX never go beyond the confines of your PC, trimming waiting times and minimising security risks inherent to transmitting data to an external server.
ChatRTX is still in the early stages of development without a v1.0 to its name for now. However, even these ‘demo’ versions are plenty useful and show promise.
RTX Video
As someone with a 4K HDR display, a feature I use almost every day is ‘RTX Video’. You’ll find it ready-to-go in the Nvidia App’s ‘System’ section, under the ‘Video’ tab. This feature is actually two-in-one, made up of ‘Video Super Resolution’ and ‘High Dynamic Range’. The former uses AI to upscale the resolution of video content while also reducing compression artifacts. Meanwhile, the latter converts SDR video to an HDR colour space like the RTX HDR game filter which I highly recommend checking out.
You can practically set and forget Video Super Resolution but it won’t activate unless you’re watching content via VLC Media Player or a supported web browser like Google Chrome or Microsoft Edge. I personally find that the ‘Auto’ quality setting works just fine, but there are four presets available if you want to better control how much it leverages your GPU versus the fidelity of the final output.
High Dynamic Range requires some trial-and-error testing to hit that sweet spot for your eyes. Once you have your settings locked in, though, it can transform SDR content in a manner that’s literally a sight to behold. To be clear, the results won’t ever replace a true HDR experience, but it comes close enough that it’s a true nice-to-have.
Nvidia Broadcast
GeForce RTX GPUs are already great to stream on thanks to their support for the NVENC AV1 video encoder but they can also improve the quality of your camera and microphone setup via Nvidia Broadcast. Through AI enhancements, this application provides echo and noise-cancelling functions to your mic and can unlock new features for your webcam.
As someone that shares office space, I value privacy when it comes to video calls. Through Nvidia Broadcast, I’m able to quickly apply a background blur or replace my backdrop entirely with an image or video of my choice.
Other effects include ‘Auto Frame’ which ensures your face stays front and centre of the camera, allowing one to fidget and move to your heart’s content. You can run this and several other options simultaneously and easily configure them through Nvidia Broadcast.
Nvidia Broadcast will receive a big upgrade closer to the launch of GeForce RTX 50 Series. This new version will include a ‘Studio Voice’ microphone effect, virtual key light, and even a streaming AI assistant.
Accelerate Creativity
The number of applications that leverage GeForce RTX hardware is growing by the day. I’ll cover some of the more mainstream examples below, but Nvidia provides a comprehensive list of other software that benefits from the likes of CUDA, Tensor, and ray tracing cores.
Since these features are all local to your PC, this not only makes your data more secure as it never leaves your system, but cuts down on time and expense. Unlike cloud-based AI, there’s no waiting in a digital queue to upload your content, dependency on broadband speeds and connection strength, or risk to your privacy as you trust a third-party to handle your information. After all, who wants to endure gruelling wait times for massive projects, let alone pay for the bandwidth to do so?
Adobe Creative Cloud
There are myriad ways that GeForce RTX graphics cards improve the performance of the Adobe Creative Cloud suite, through the power of their CUDA and Tensor Cores.
Important as raw graphics horsepower is for Premiere Pro both for a smooth editing experience and final render, there are several advantages to running the application with an Nvidia GPU. For example, with GeForce RTX in tow you now have access to NVENC encoding and NVDEC decoding which respectively speed up exporting and previews of high-resolution content.
Tensor Cores come in clutch to speed up the editing process, with features such as ‘Scene Edit Detection’ which will speedily detect cuts in a source file and split them accordingly into individual clips. Other useful AI tools include colour matching, speech enhancement, and ‘Auto Reframe’, the latter of which makes keeping track of subject across horizontal and vertical aspect ratios much easier.
Photoshop offers more than 30 GPU-accelerated features which GeForce RTX graphics cards can make operate more smoothly and quickly.
Naturally, AI can assist here too. Combatting the pains that come with low-resolution assets, ‘Super Resolution’ can deliver higher-fidelity images than standard upscaling techniques. ‘Neural Filters’ can produce similarly impressive results. Examples like ‘Photo Restoration’ can greatly speed up the process of restoring old photographs to their former splendour.
GeForce RTX graphics cards’ CUDA and Tensor Cores also benefit other applications in suite, such as After Effects, Illustrator, Lightroom, and more.
Blender
3D modelling can be massively taxing on your graphics card, but GeForce RTX cards have a few tricks up their sleeves to alleviate the pressure and save time.
GPU-accelerated motion blur is not only much faster to apply than manual workarounds, it also results in more accurate, higher-quality end results. CUDA cores make exporting final projects all the faster.
Ray-traced rendering via the CPU is possible but is tediously slow relative to a GPU. GeForce RTX graphics cards are all the faster thanks to their built-in RT cores, which offer advanced ray tracing features and performance. Better still, they can work with the Tensor Cores to provide AI-powered ‘OptiX Denoising’ for a cleaner final image.
DaVinci Resolve
DaVinci Resolve is one of the most-versatile and powerful editing suites on the market. Naturally, performant graphics cards like GeForce RTX Series GPUs are a must for unlocking its full potential.
In addition to support for NVENC AV1 encoding and NVDEC decoding, DaVinci Resolve includes several tools that enhance projects through AI.
‘RTX Video Super Resolution’ removes video artifacts and can upscale lower-resolution source content, from 1080p to 4K for example. Meanwhile, ‘RTX Video HDR’ can bring further vibrancy to SDR content by translating colours to a wider gamut.
More advanced features include face recognition and the auto-tagging of clips, in addition to the likes of ‘SpeedWarp’ for smooth slow motion. Through this handful of tools and more, there’s no question how GeForce RTX graphics cards make for a more efficient edit.
AI Benchmarked
It’s all well and good to understand what Tensor Cores are and how they can help, but getting the performance meat on the bones illustrates their true utility in boosting performance in applications discussed above. To that end, I’ve benchmarked the AI chops and relevant applications on two recent Nvidia cards: GeForce RTX 4090 and GeForce RTX 3070, along with some comparisons to running the same workloads on leading CPU alone.
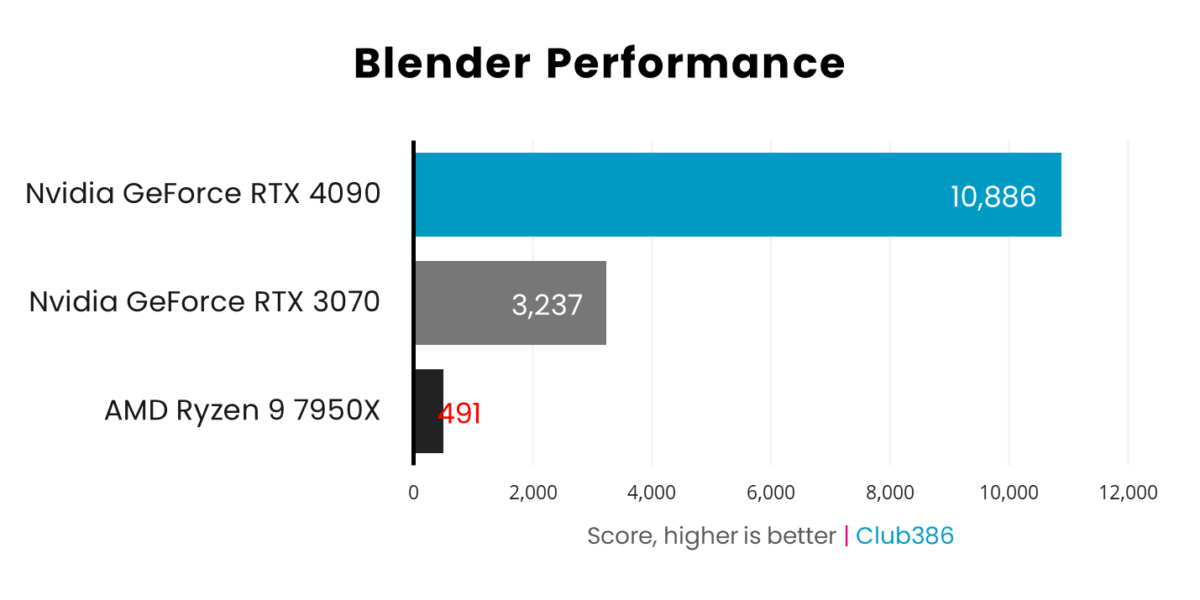
These scores highlight the stark difference in performance between running multimedia applications on CPUs and GeForce GPUs. For example, an RTX 3070 produces 6.6x the performance, while RTX 4090 is a whopping 22x quicker.
Putting this in time context, a rendering project that takes an hour on the 16-core, 32-thread AMD Ryzen 9 7950X CPU ought to take less than three minutes on the RTX 4090. When time is money and projects need to be out the door, there’s only one clear winner.
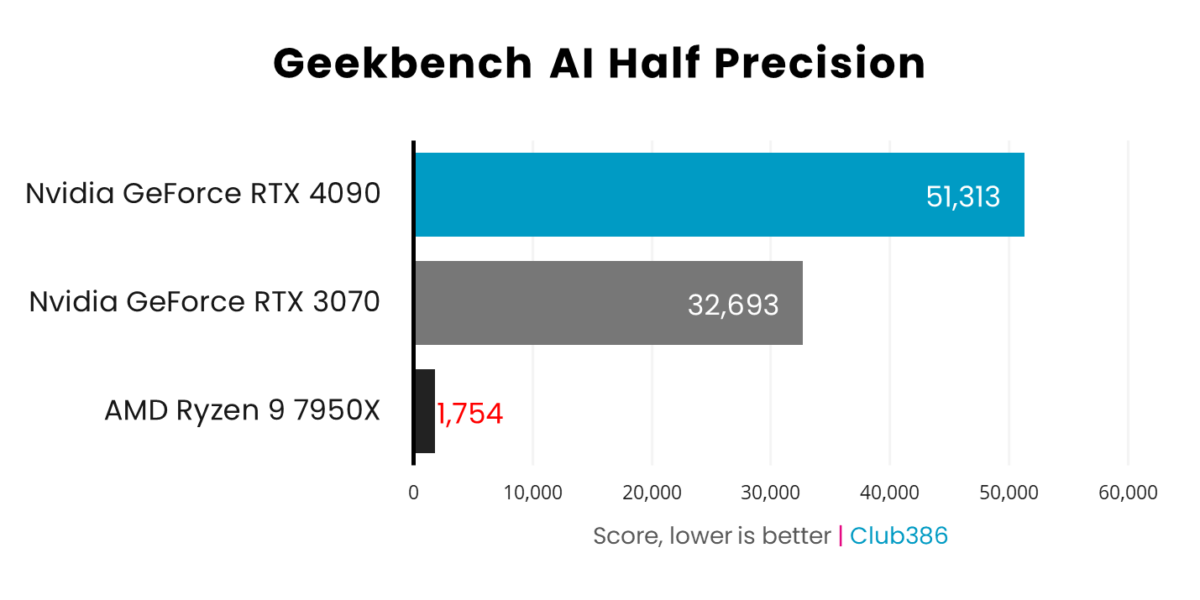
The Geekbench benchmark calculates the AI capability of accelerators. Using the popular half-precision setting – also known as 16-bit – we see a similar story to the first. Here, the speed-up is almost 30x from Ryzen 9 7950X to RTX 4090.
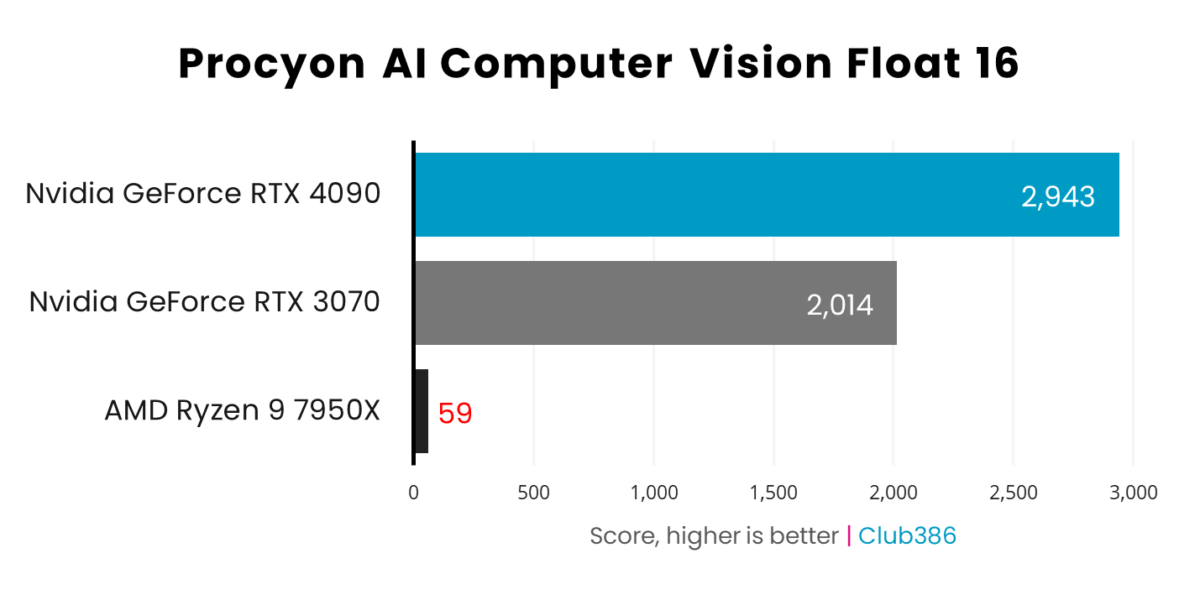
This benchmark runs through numerous neural network models – visual recognition, image classification, object detection, super resolution, etc. – and evaluates performance. The faster these networks can run tasks, the quicker you get meaningful results.
Looking at the broad church of uses cases, we see a 50x improvement for RTX 4090 over Ryzen 9 7950X, while RTX 3070 is 34x faster.
Of course, one expects GPUs and Tensor Cores to be better at these parallelisable tasks, but it remains eye opening how much more quickly they’re able to provide results that lead to a good experience.
Want image-to-text generation that takes seconds and not minutes? Run it on a class-leading RTX. Need a large-language model to provide a near-instant response to any query you can think of? Don’t use CPUs.
Knowing that RTX cards are simply better than CPUs in many AI tasks, a good question to answer is how different solutions perform against one another when looking at acceleration built into applications. I can do this by examining RTX 3070 and RTX 4090 in the latest DaVinci Resolve AI tests that are useful for tasks like video stabilisation, face refinement, audio translation, smart refresh, et al.
DaVinci Resolve | RTX 3070 fps | RTX 4090 fps |
---|---|---|
Super Scale | 30.62 | 84.91 |
Face Refinement | 17.67 | 30.62 |
Relight | 8.9 | 16.01 |
Magic Mask Tracking | 28.77 | 59.03 |
Optical Flow | 6.11 | 15.07 |
Run Time Reframe | 1391 | 2707 |
Picking six variables shows speed-ups of anything between 1.8x through to 2.77x. You’d expect as much, really, given the RTX 4090’s intrinsic might, yet it’s good to know there’s genuine scaling between RTX GPUs for applications that matter to creators.
Another key benefit of powerful GPUs within your system is local processing of text-generation benchmarks derived from large-language models. Think of these as scaled-down ChatGPT running on your PC, rather than in the cloud. A faster RTX card helps, but here I tell you by how much.
Procyon LLM | RTX 3070 score | RTX 4090 score |
---|---|---|
Phi 3.5 | 2,557 | 4,793 |
Mistral 7B | 2,198 | 5,011 |
Llama 3.1 | 2,005 | 4,745 |
Llama 2 | 0 | 4,963 |
First thing’s first. Llama 2 doesn’t run on the RTX 3070 due to insufficient RAM; the card’s 8GB is simply not enough to store all required parameters. Nevertheless, looking to other models, you can expect between 1.9x to 2.4x more performance when switching out the behemoth that is RTX 4090.
The humble CPU, meanwhile, is so outgunned in these tasks that it provides practically meaningless results in DaVinci Resolve – what takes seconds takes multiple minutes – and simply cannot run mid-sized LLMs without stumbling so much that it’s verging on unusable.
Conclusion
Released in 2018 with the GeForce RTX 20 Series of GPUs, Nvidia devoted meaningful die space to new-fangled Tensor Cores. The prevailing thought of gamers at that time was one of bemusement, wondering why the focus on a technology with no immediate pay-off. Certainly, the Tensor Core die ought to be saved for traditional shader units for more rasterisation performance, right?
Fast forwarding seven years brings fresh perspective. What was once a fledging initiative has turned into arguably the most important component of modern RTX cards. Without visionary Tensor Cores, let me remind you, they’d be no DLSS – improving base framerates by factors of 5x and more in certain games – or innate ability to fundamentally accelerate burgeoning AI workloads that are becoming pervasive in everyday applications.
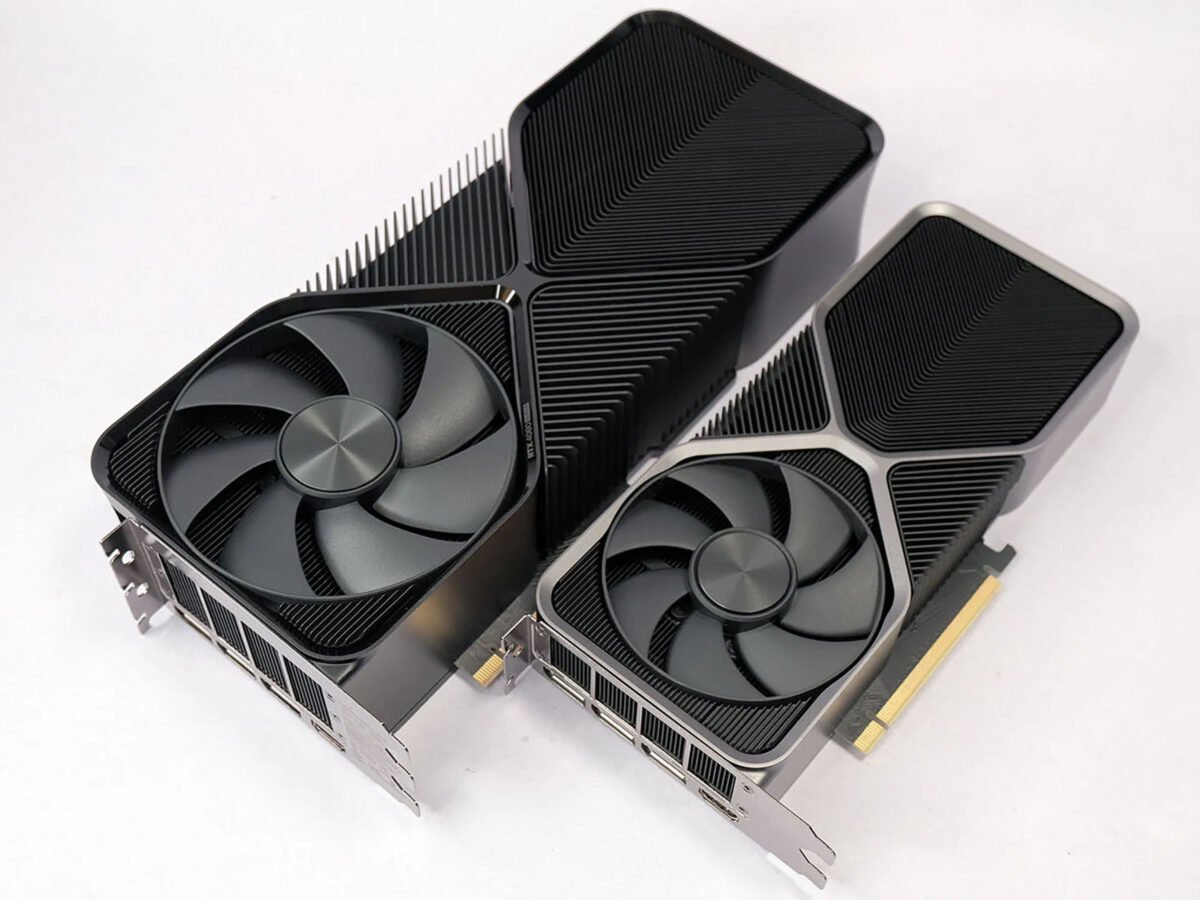
Nvidia GeForce RTX Tensor Cores are a boon for many workflows and provide quality-of-life improvements for everyday PC usage. Popular creative applications such as Adobe Photoshop, Premier Pro, and DaVinci Resolve all now include options to use RTX GPUs for processing-heavy tasks, turning completion time from hours to minutes in some cases. Applications such as Nvidia RTX Video and Broadcast, meanwhile, lend a helping hand for improving video and suppressing noise.
Putting performance into context, running applications on class-leading GPUs is comfortably 10x faster than on a premium CPU. The lead extends to over 30x in corner cases, highlighting that if your chosen app can use GPU acceleration, you really should turn it on.
Furthermore, the AI capabilities baked into RTX graphics cards allow them to run large-language models locally, omitting the need to use cloud-native rendering and pursuant latency and security concerns.
Nvidia GeForce RTX graphics cards are more than painters of pretty pixels. Their robust chops pave the way for radically reducing the time taken for complicated creative and emerging AI workflows, all the while providing quality-of-life features that you didn’t know you needed.